3.8: Adoption Trends
( \newcommand{\kernel}{\mathrm{null}\,}\)
- Discuss people analytics adoption trends
Deloitte’s 2018 Global Human Capital Trends survey results indicate that “the use of workforce data to analyze, predict, and improve performance has exploded in practice and importance over the last few years,” with 84% of respondents rating people analytics as important or very important.[1] According to Bersin by Deloitte’s High-Impact People Analytics Study, 69% of large organizations have formed a people analytics team and are building an associated database. Bersin notes that in prior years, the percentage was a relatively constant 10–15%.
In a related article titled “People Analytics: Here With A Vengeance,” Bersin highlights key study findings that illustrate the accelerating pace of adoption:[2]
- People Analytics has grown up—it is now an established discipline in business.
- As the statistics cited above illustrate, CEOs and CHROs now understand the link between people analytics and business performance. There’s also a recognition that people analytics can do so much more than simply “cost-justify HR investments;” indeed, people analytics supports effective action on a range of high-priority issues including diversity, gender pay equity, skills gaps and retention rates.
- The problems of data quality, integration, and integrity are being addressed.
- Businesses report significant progress in addressing data quality and integrity issues that have historically been an obstacle. Specifically, 39% of respondents reported having “very good” or “good” quality data to support people-related decisions and 31% reported an understanding of “best-in-class” people analytics.
- Companies are greatly expanding the type, nature, and level of data to analyze.
- In addition to traditional data regarding candidates, pay, performance, training and other lifecycle data, organizations are compiling an extensive amount of data—increasingly in real-time—that reflects employee engagement, sentiment, behavior, well-being and other data that can be used to profile high-performers, identify patterns of behavior and inform decisions and interventions.
- Data and analytics literacy has become an imperative for HR professionals.
- Most business managers recognize that data is key to their success. They won’t listen to unsubstantiated claims of bias or culture issues. What they’re seeking is the data to prove what’s not working and data-driven recommendations for improvement.
- AI and Machine Learning have arrived – and People Analytics teams are using these algorithms to partner with the business
- Bersin observes that “we used to think the secret to productivity at work was ‘skills.’ Now, through the use of machine learning, we can understand that the secret is also ‘behaviors,’ ‘habits,’ and ‘patterns’ that highly successful people adopt. Many of these are unconscious [and can’t be observed] by the experts, but can be analyzed and understood by software.”
For a visual view of adoption, refer to Figure 1, which illustrates people analytics adoption based on an organization’s level of maturity or implementation of people analytics. The model, created by Bersin, categorizes adoption based on four progressive levels of “maturity,” ranging from Level 1 through 4. In Level 1, which represents 14% of the organizations surveyed, data is not considered a value-driver and decision-making is still driven by intuition, experience and precedence.[3] In Level 2, representing 69% of the organizations surveyed, there is a focus on building people analytics capabilities and a consistent use of analytic tools—primarily in support of HR. In Level 3, approximately 15% of those surveyed, organizations are using advanced tools and technologies to collect, integrate and analyze data relevant to the business broadly. In Level 4, representing maturity, organizations are using real-time, AI-aided tools and technologies to college, integrate and analyze data and people analytics is integral to business, HR and management decisions; 2% of the organizations surveyed are operating at this level.

In their “Rise of Analytics” report, LinkedIn notes that people analytics adoption is concentrated in specific industries (see Figure 2). The overall adoption of HR-focused analytics teams is still relatively low, with only 22% of companies using HR analytics and 11% with an HR analytics role.
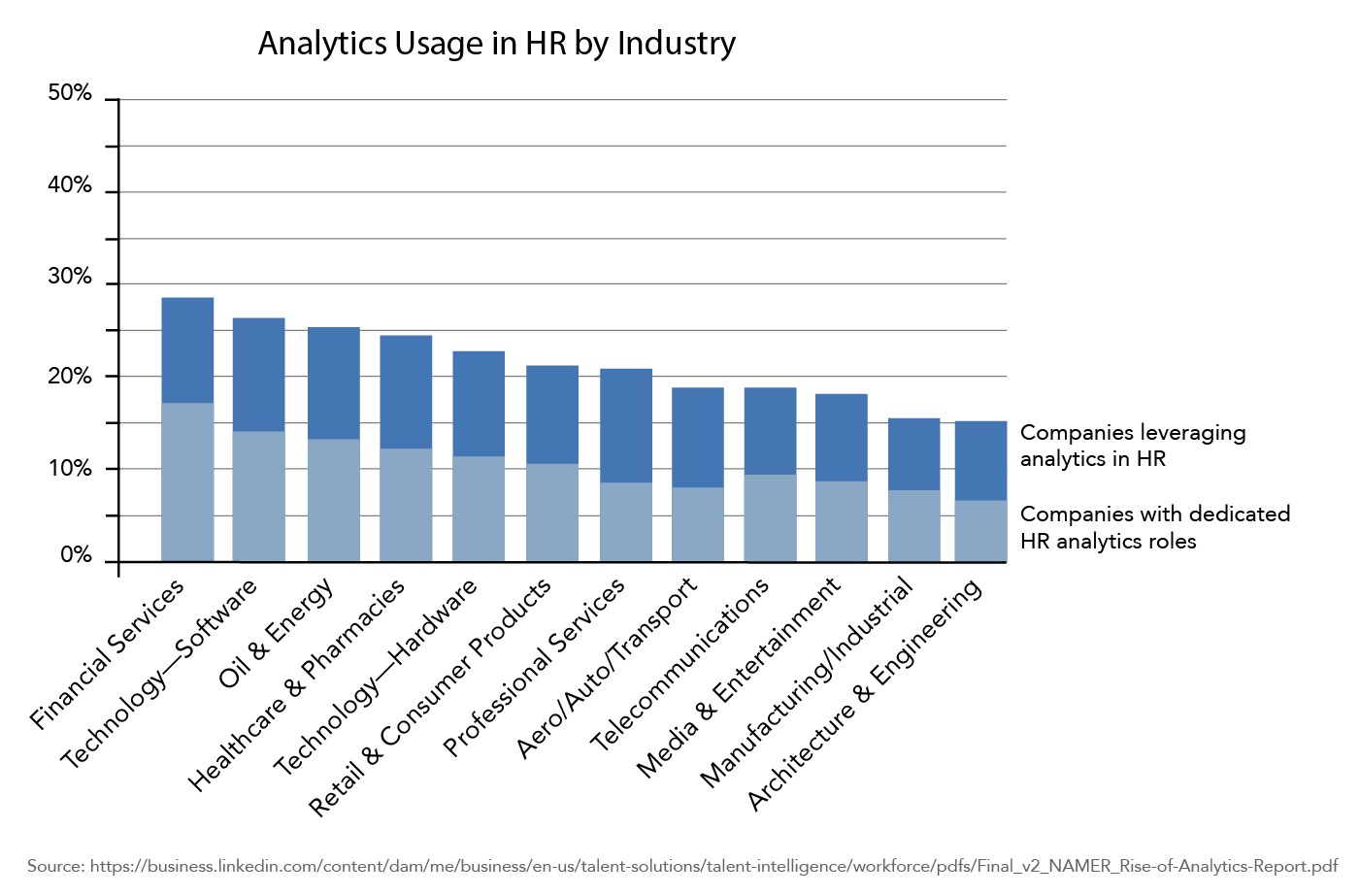
Industry | Companies leveraging analytics in HR | Companies with dedicated HR analytic roles |
---|---|---|
Financial Services | 11% | 16% |
Technology – Software | 11% | 14% |
Oil and Energy | 11% | 12% |
Healthcare & Pharmacies | 12% | 12% |
Technology – Hardware | 10% | 11% |
Retail & Consumer Products | 10% | 11% |
Professional Services | 11% | 8% |
Aero/Auto/Transport | 10% | 7% |
Telecommunications | 10% | 9% |
Media & Entertainment | 8% | 8% |
Manufacturing/Industrial | 7% | 7% |
Architecture & Engineering | 8% | 6% |
- Abbatiello, Anthony, Dimple Agarwal, Josh Bersin, Gaurav Lahiri, Jeff Schwartz, and Erica Volini. "2018 Deloitte Global Human Capital Trends." Deloitte Insights. 2018. Accessed August 06, 2019. ↵
- Bersin, Josh. "People Analytics: Here With A Vengeance." JOSH BERSIN. December 19, 2017. Accessed August 06, 2019. ↵
- Bersin, Deloitte Consulting LLP. "Figure 1. People Analytics Maturity Model." 2017. ↵
Contributors and Attributions
- Untitled. Authored by: You X Ventures. Provided by: Unsplash. Located at: https://unsplash.com/photos/Oalh2MojUuk. License: CC0: No Rights Reserved. License Terms: Unsplash License